What are we trying to achieve ?
Extreme-environment materials require accurate performance (mechanical response and lifetime) predicting capabilities that will only be achieved if historical effects are accounted for. The proposed synergistic experimental and modeling approach will fill this knowledge gap by providing a fundamental understanding of how thermally and mechanically-induced solid-state transformations are affected by strain memory. This will help formulate a unique two-surface crystal-plasticity model that will be at the cornerstone of improved predictive capabilities for the in-service thermo-mechanical responses of advanced materials with solid-state transformations. Furthermore, a deep-learning model, viz., a graph neural network, will be trained using the full-field simulation results and is expected to provide quick texture-dependent (orientation and arrangement) insights onto strain-memory effects. The project will, therefore, brings forth accurate and fast predictions of strain-memory effects that will contribute to a long-term engineering solution for materials with solid-state transformation exposed to complex solicitations and microstructures, like additive-manufacturing materials that are heavily textured and display out-of-equilibrium micro-structures.
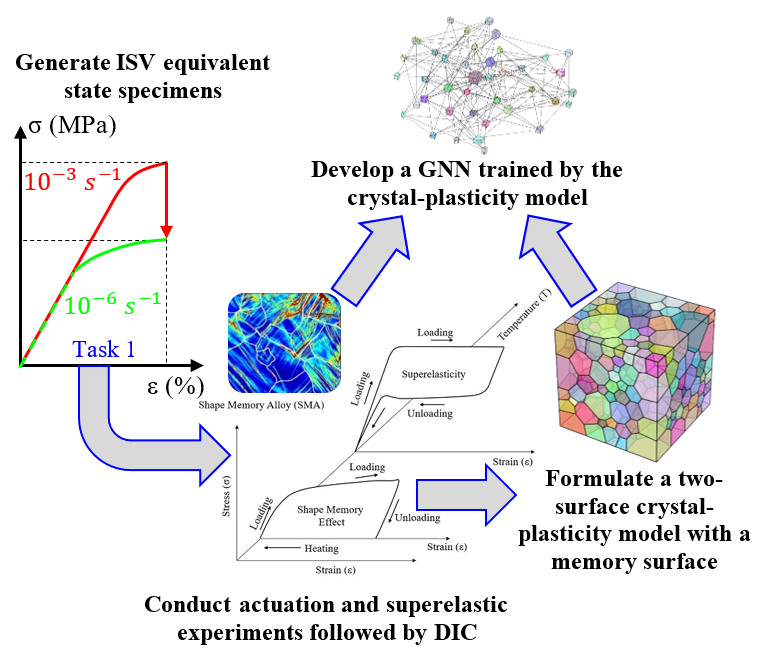
Here a the grant we obtained to work on this topic:
- NSF : Strain-memory Effects on Solid-state Transformation (PI : J.-B. le Graverend, Co-PI : D. Hajovski & S. Ji ; $566,858 ; May 2024-April 2027)
Abstract
Extreme-environment materials require accurate performance (mechanical response and lifetime) predicting capabilities that will only be achieved by accounting for strain-memory effects. The synergistic experimental and modeling approach in this project will fill this knowledge gap by providing a fundamental understanding on how thermally- (actuation) and mechanically-induced (superelasticity) solid-state transformations are affected by the history of deformations. This will help formulate a unique two-surface crystal-plasticity model that will be at the cornerstone of improved predictive capabilities for the in-service thermo-mechanical responses of advanced materials with solid-state transformations. However, though crystal-plasticity modeling has many advantages, full-field simulations are computationally expensive and call for faster computational techniques while maintaining texture sensitivity. Hence, a graph neural network (GNN) will also be developed and trained with full-field simulations from crystal-plasticity modeling. The project will, therefore, bring forth accurate and fast predictions of strain-memory effects that will contribute to a long-term engineering solution for materials with solid-state transformation exposed to complex solicitations and microstructures.
Where can that be used?
Martensitic transformation is key in structural materials, like steel, in nuclear power generation, and also in functional materials, like shape memory alloys, in aerospace. Understanding how martensitic transformation is affected by strain memory will therefore directly benefit the aerospace, microelectronics, automotive, and power generation industries, for any improvement in the pre-diction of mechanical performance will bring about cost savings and better life-cycle management. In addition, evidence of “martensitic”-type transformation has been found in many ferrous and non-ferrous alloys and in several oxides and intermetallic compounds. So, it is foreseen that the fundamental insights gained by the project would also be applicable to other systems, such as age-hardenable ceramics, martensitic reactions in ionic crystals, and typical phase changes in crystals of rare gases.
Who is involved?
J.-B. le Graverend (PI)
S. Ji (Co-PI)
D. Hajovsky (Co-PI)
Hope Elmer (Ph.D. Student)
Adrien Cassagne (Ph.D. Student)
Who is funding?
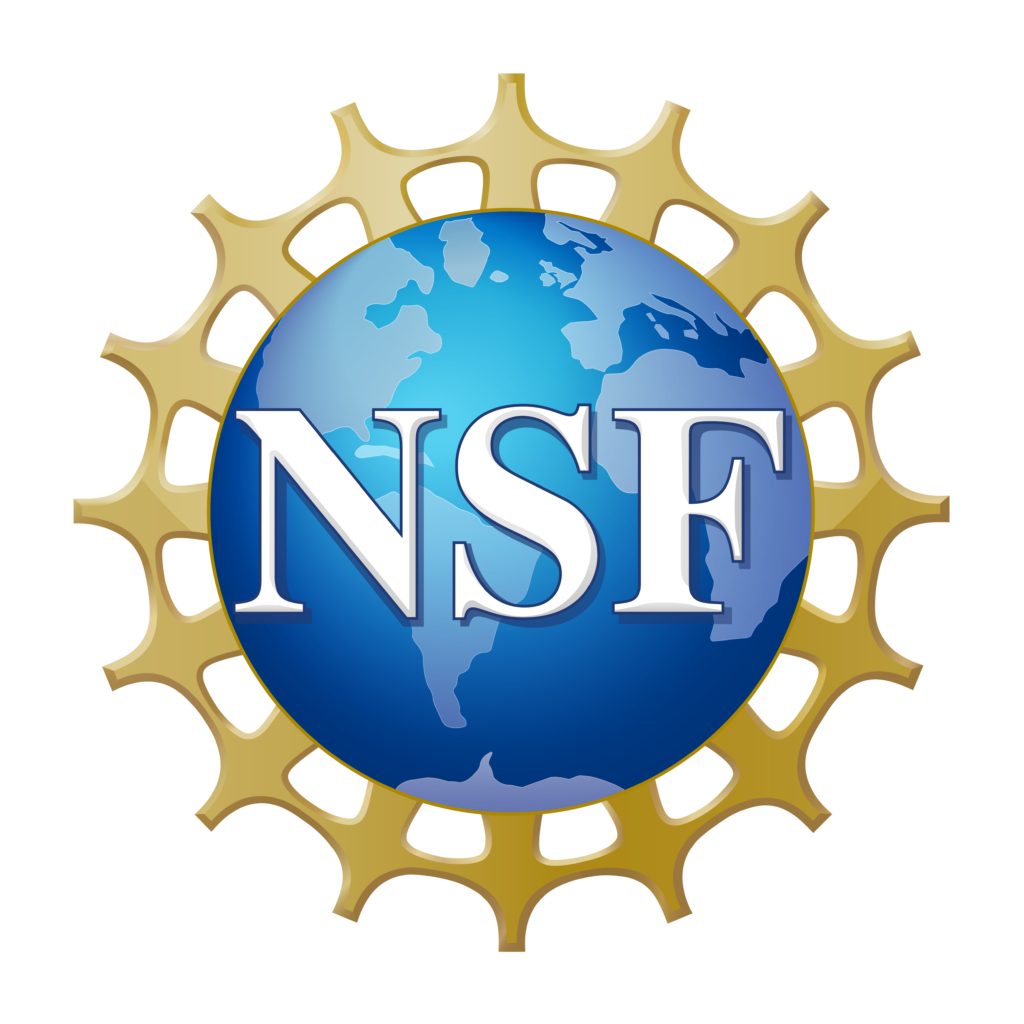
National Science Fondation
Publications and Conferences
Coming